Simple random analysis
These formulas are appropriate for data collected via both simple random and systematic surveys with parallel transects (Simple Random Sampling). Each transects provides a single local estimate of fish density. Calculations of means and variance follow the standard methodology and assume that the randomly selected observations are independent and identically distributed:
We can compute the average density (
) and variance (s2ρ) from the average density for each transect i (
ρi) over all n transects:
|
|
[33] |
|
|
[34] |
The standard error for the estimate of the average abundance per transect
(SE(
) is:
|
|
[35] |
As discussed earlier, expansion of an estimate of average density per unit area (ρa) or per unit volume (ρv) to an estimate of the total population (N) requires additional knowledge and assumptions. Assuming the transects are representative of the whole area (A) and that this area is known absolutely (has no variance), the expansion is straight forward:
|
|
[36] |
where A is given in units of total area or total volume, and
is in units of density per area or volume.
The corresponding standard error of the abundance estimate (SE(N)) would be:
|
|
[37] |
Stratified analysis
These formulas are appropriate for surveys with systematic samples nested within strata (Stratified Sampling). The approach is very similar to the calculations used for simple random sampling, but individual estimates are calculated for each stratum and then merged based on the relative size of each stratum.
We can compute the average density (
h)and between transect variance (s2ρh) within each stratum h:
|
|
[38] |
|
|
[39] |
where:
nh is the number of transects in strata h, and;
ρhi is average density on transect i in strata h.
The global mean for the stratified estimate (
str) is:
|
|
[40] |
where:
L is the total number of strata;
A is the total area of all strata A=A1+A2+...+AL
Ah is the total area of each stratum h, and;
h is the average density within each stratum h.
The corresponding standard error for the stratified estimate (SE(
str)) is:
|
|
[41] |
where:
nh is the number of transects in each stratum h;
s2ρh is the between transect variance in average abundance for strata h.
As above, assuming the transect are representative of each strata and that the area of each strata is known absolutely (has no variance), the expansion to total population is identical to the simple random survey calculations (Equations 36 and 37).
Cluster sampling
Cluster sampling may be used for systematic or random parallel transects or for zig-zag transects using only parallel zigs OR parallel zags.
Cluster sampling is an appropriate design and analysis method to consider for acoustics as clusters of observations are typically taken along a transect and not as, say, independent 1-minute sample units randomly scattered throughout the population. The clustered nature of the samples often requires that additional attention be paid to the type of analysis used so that the most can be made from the number of samples collected. A major advantage of this method is that it will weigh estimates according to transect length. Since transect lengths are seldom identical, this is the recommended method for acoustics surveys in general when geostatistics is not being used (see below).
In an acoustic example of cluster sampling:
Transects are clusters, and;
Horizontal bins are elements within clusters.
The first step is to compute an aggregate density estimate Pi across all the elements in each cluster i as follows:
|
|
[42] |
where:
mi is the number of elements (bins) in cluster (transect) i;
ρj is density in horizontal bin j (#m-2);
Notice that Pi is also in units # m-2, but this is misleading as it Pi represents the sum of all densities and is therefore a function of the number of bins. If we multiply this estimate by the average area per bin we would get total number per transect, which is typically used in textbook presenting cluster analysis, but we leave that extra bit of calculation out here as, in the end, it cancels out.
We can compute the average density:
|
|
[43] |
where:
n is the number of clusters (transects) in the sample;
Pi is the aggregate density observed in cluster i, and;
mi is the number of elements (bins) in cluster i, with i = 1,…, n.
The cluster variance (s2clu) and the standard error of the estimated average number per bin (SE(
)) may then be found:
|
|
[44] |
|
|
[45] |
where:
Pi is the agregate density in cluster i;
is the average number per bin over all clusters;
mi is the number of elements (bins) in cluster i, i = 1,…, n;
n is the number of clusters in the simple random sample; and
is the estimated average number of elements (bins) per cluster (transect), such that
|
|
[46] |
Cluster sampling estimates may be expanded to total population abundance (N) by simply multiplying average density by area:
|
|
[47] |
where:
A is the total area;
is the average density (#m-2 area or #m-3 volume).
The standard error of the population abundance is:
|
|
[48] |
where, again, SE(
) is the standard error of the estimate mean density derived from the cluster sampling method described above.
Geostatistics
Geostatistics is an appropriate approach to apply to data collected with zig-zag, systematic parallel, stratified parallel, or random parallel transect designs.
This section provides a brief overview of the theory of geostatistics. To read further about geostatistical theory and application of these techniques, readers are referred to Rivoirard et al. (2000), Kaluzny et al. (1998), or Goovaerts (1997).
If there is reason to believe that the distribution follows some definable stochastic process, one would use a geostatistical procedure for obtaining the estimates.
A variogram is used to examine correlation among sub-elements (horizontal bins) of transects. The variogram takes the form:
|
|
[49] |
where:
ρ is an observation (e.g. density) referenced to its location si=[lat,lon]i;
h is a distance vector separating theobservations such that si – sj = h, and;
N(h) is the number of pairs of data locations that are a distance h apart.
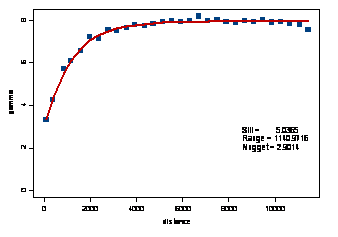
Figure 34. Empirical variogram data gathered on Sv using a 120 kHz echosounder averaged over the water column (<100 m deep) and fit with an exponential theoretical model with sill=5.04, effective range=3300 m, and nugget=2.90. As distance increases between two points the variance of their difference, as denoted by the variogram, increases and then plateaus out at roughly the global or maximum variance value. This global variance (or sill) minus the variogram estimate results in the perhaps more familiar correlation curve showing decreasing correlation with distance.
The resultant empirical variogram (example Fig. 34) is then fit with a theoretical model with the components:
Range: the distance at which there is no correlation in the data;
Sill: representative of the maximum level of variance in data, and;
Nugget: the level of measurement error or microscale processes near h=0.
If correlation exists, the ordinary kriging predictor may be used to predict the distribution of Sv or density over the entire sampling frame. The ordinary kriging predictor is unbiased and fairly stable under different predictive conditions (Cressie 1993). The prediction of ρ at the point s* is:
|
|
[50] |
with prediction variance:
|
|
[51] |
where C(0) is the variance at lag zero, n(s*) is the number of observations in a neighborhood of s*, m is the Lagrange multiplier, and where :
The vector of weights is given by:
|
|
[52] |
As seen here, these weights (λ) are based on a function of the variance-covariance matrix k between the observations and the point being estimated ρ(s*) and the variance-covariance matrix K between each of the observations. These covariances may be computed using the variogram and the relation C(h)=C(0)-γ(h) when the variation at lag zero, C(0), estimated by the sill, is well defined.